In the fast-paced world of data technology, one sector that's creating significant buzz is generative artificial intelligence (AI). This groundbreaking innovation can create content, such as music, art, or even literature, that is indistinguishable from that produced by human creators. But the power of generative AI's output is only as good as the data you feed it.
For IT managers and professionals looking to harness the potential of generative AI, data management is not just about organization; it's the fundamental building block that can significantly impact the AI's efficacy. Here, we'll explore some essential data management practices that can amplify generative AI systems' capabilities.
Understand the Unique Data Needs of Your AI

Generative AI models, such as those used for natural language processing (NLP) or computer vision, require vast and diverse datasets to learn. The first step is to understand deeply what kind of data your AI system needs. For example, a language model like GPT-3 would need a corpus representing various writing styles, topics, and levels of expertise. It’s not just the variety of data that's crucial; the quality is equally important. Ensure that the input data is clean, labeled appropriately, and free from biases.
Gather and Categorize Data Thoughtfully
The quality of the model's output is directly related to the quality and representativeness of the input data. Be meticulous in your data-gathering process. This may involve scraping a wide range of websites for language analysis or curating a collection of thousands of images for computer vision. Categorize your data sets clearly, marking the data with attributes and metadata to help the training process and reduce noise.
Enforce Data Security and Privacy
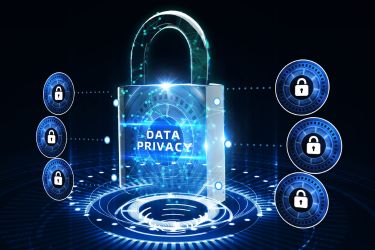
With significant data comes great responsibility. Data breaches and unauthorized access can not only lead to legal repercussions but can also undermine public trust. Implement stringent security protocols to protect the privacy of your datasets. Anonymize personal data, encrypt sensitive information at rest and in transit, and restrict access on a need-to-know basis.
Leverage the Power of Big Data Solutions
Generative AI requires extensive computational resources and storage capacity. Big data solutions like Hadoop or Spark can handle the vast volume of data and the complex computations that come with training large AI models. These platforms also provide the scalability needed for growing datasets and can handle diverse data types.
Document and Version Your Data
Maintain clear and thorough documentation of your data to provide context for your AI training. This includes the data's origin, the preprocessing steps applied, and any assumptions made during the preparation. Additionally, version control allows you to track changes to the dataset over time, which is crucial for reproducibility and troubleshooting.
Integrate Data Management with Model Training
Data management doesn't end when the data is collected. It's integral throughout the model's lifecycle. An integrated approach that links data management to model training means that the AI system can adapt as new, verified data is added to the dataset. This process requires developing a systematic approach to retraining models with updated data.
Monitor Data Quality and Model Performance Continuously
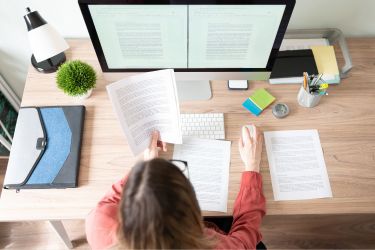
Data quality degrades over time, and the performance of your AI models will, too, if not maintained. Establish automated systems for regular data quality checks and monitor the performance of your AI models. Use feedback loops to ensure the model’s output aligns with the data quality and the application's objectives.
Plan for Data Growth and Model Evolution
Plan for scale and model evolution from the outset. Your data management strategy should be flexible enough to handle the growth of data and the adoption of new machine-learning techniques. Regularly assess the maturity of your data management practices and evolve alongside the field of AI to stay ahead.
Generative AI Data Management for IT Professionals
Data is the lifeblood of AI, and effective management of this data can make or break your generative AI projects. By understanding your AI's data needs, gathering and categorizing your data thoughtfully, enforcing security and privacy measures, and integrating data management with model training, you can lay a solid foundation for generative AI's success and ethical use. With these practices in place and adopting data security management best practices, you can harness the true power of AI to create remarkable, human-like outputs.